Haotian Cui, Ph.D.
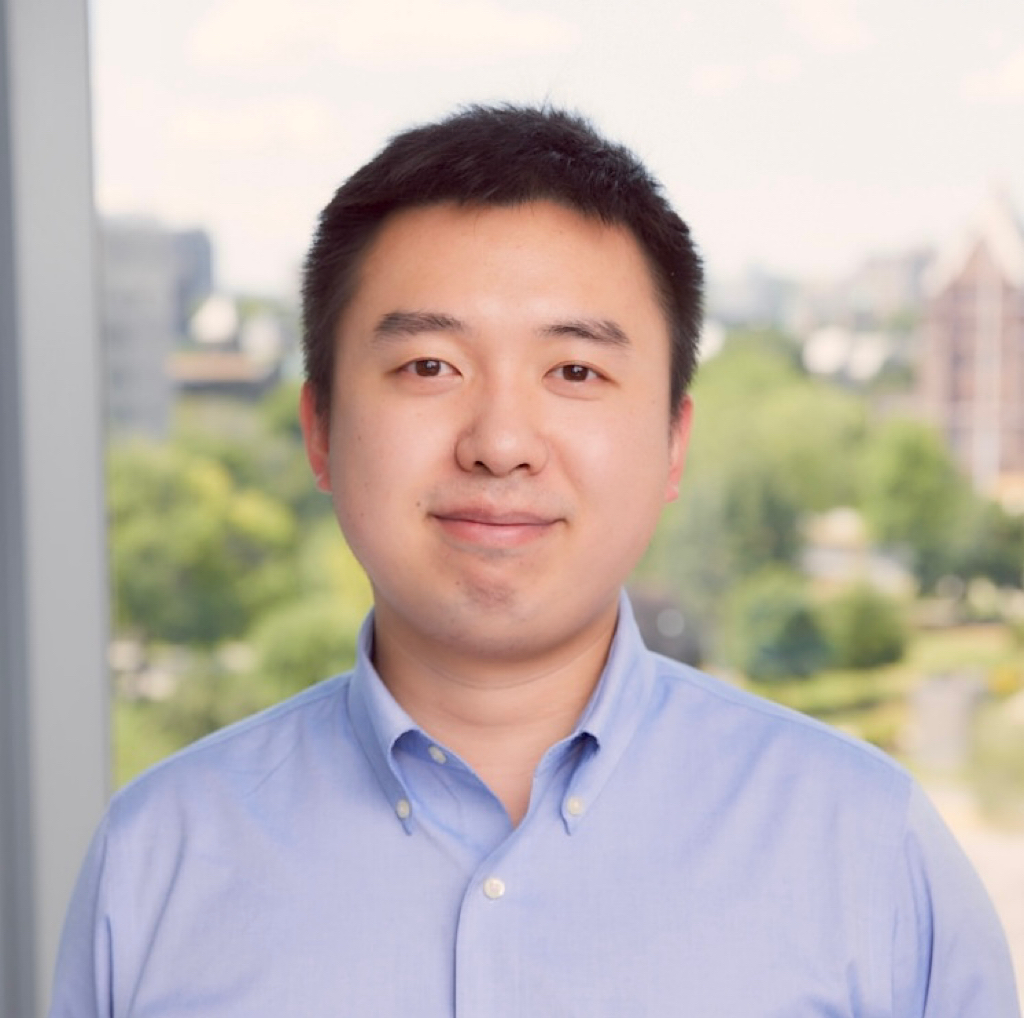
I am a researcher specializing in machine learning, genomics, and drug discovery. My work focuses on developing large-scale self-supervised models to enable biological insights and therapeutic discoveries. I am passionate about building foundation models for single-cell omics and molecular biology, integrating generative AI with experimental pipelines to accelerate biomedical breakthroughs.
I completed my Ph.D. in Computer Science at University of Toronto (2019-2024) under Prof. Bo Wang, where I pioneered scGPT, one of the first generative foundation models for single-cell multi-omics. I also led the development of LUMI-lab, an AI-driven autonomous lab for mRNA therapeutics.
Research Interests: Foundation model, Machine Learning, Genomics, Drug Discovery
selected publications
- Towards Multimodal Foundation Models in Molecular Cell BiologyNatureForthcoming. Perspective on multimodal foundation models across the central dogma. , 2025
- bioRxivscGPT-spatial: Continual Pretraining of Single-Cell Foundation Model for Spatial TranscriptomicsbioRxiv, 2025
- bioRxivMethylGPT: a foundation model for the DNA methylomebioRxiv, 2024
- Nature CommunicationsAGILE platform: a deep learning powered approach to accelerate LNP development for mRNA deliveryNature Communications, 2024
- Genome BiologyDeepVelo: deep learning extends RNA velocity to multi-lineage systems with cell-specific kineticsGenome Biology, 2024
- EMNLP 2022CodeExp: Explanatory Code Document GenerationIn Findings of the Association for Computational Linguistics: EMNLP 2022, Dec 2022
- bioRxivscFormer: a universal representation learning approach for single-cell data using transformersbioRxiv, Dec 2022
- ICML 2022A Deep Learning Framework for Estimating Cell-specific Kinetic Rates of RNA VelocityIn The 2022 ICML Workshop on Computational Biology, Dec 2022
- Nature Biomedical EngineeringStretchable ultrasonic arrays for the three-dimensional mapping of the modulus of deep tissueNature Biomedical Engineering, Dec 2023
- DaSH 2022Execution-based Evaluation for Data Science Code Generation ModelsIn DaSH 2022, Dec 2022